Learning from Teachers’ Experiences during the Pandemic
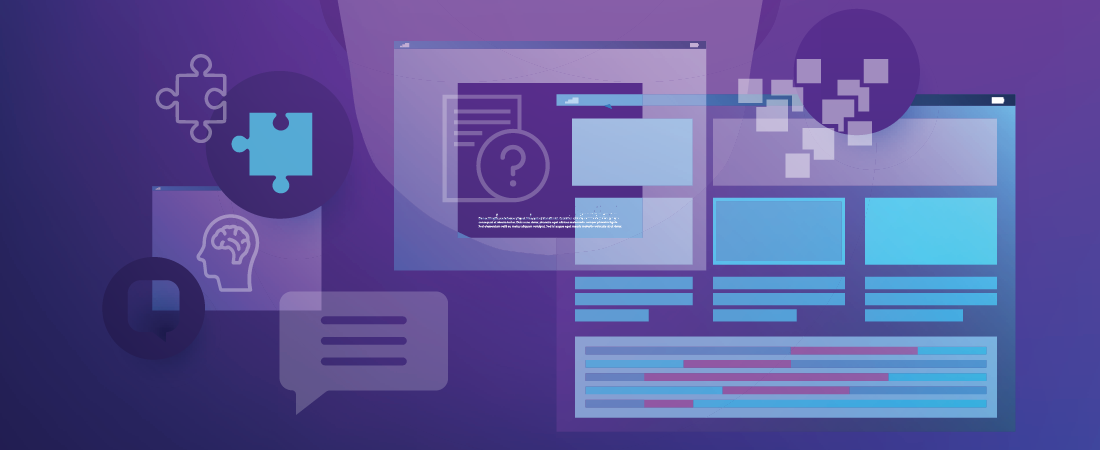
The Boston Public Schools (BPS) has more than 6,200 teachers and instructional staff members. And this past spring, as the district made an abrupt transition to remote learning due to the COVID-19 pandemic, each educator had a different set of experiences.
District leaders captured many of their stories, opinions, and observations in a set of surveys sent to every teacher, administrator, and staff member. The surveys offered staff the chance to write about effective distance learning strategies, barriers to engaging with students and families, and their professional development needs. But when it was time to analyze the data, the district ran into problems.
“We wanted to learn, what did we do well? What could we improve?” says Apryl Clarkson, the director of research for BPS. “But when we received the volume of responses, we realized that we didn’t have the capacity to review them in a timely manner.”
The stumbling block was the district’s use of open-response items. Over 1,700 respondents offered short narrative answers to some of the questions on the surveys—meaning that Clarkson and her research team would have to read and code thousands of individual responses to analyze the entire data set. And time was of the essence. Clarkson wanted to begin sharing the data with school leaders and administrators to inform supports and practices before teachers and students returned to school in the fall.
Looking for support, BPS issued a call for support from research partners. Josh Cox, an education researcher at REL Northeast & Islands at EDC, responded. Cox knew that regular research analysis methods would not turn around the data quickly enough, so he turned to a new method of data analysis—natural language processing.
An application of machine learning, natural language processing (NLP) is a computer-based method of identifying common words and phrases in a large body of text. NLP ignores articles and conjunctions and treats words with a common stem—such as teaching, teacher, and teachable—as the same. In this way, NLP can cut through some of the linguistic differences among individual writers to identify common items across all responses.
Cox thought that the method would be especially helpful in analyzing the BPS data, since many of the teachers’ responses were bound to be similar in intent if not worded identically. Yet despite its utility in sifting through large amounts of text, he says the method is still rare in education research.
“NLP is often used to comb through content on websites and in social media posts, so there is some novelty about using it to analyze surveys like those that were administered by BPS,” Cox says.
Cox began the analysis by mapping the nine survey questions to five research questions. He then wrote code in the Python programming language that would apply NLP to the data set.
Cox estimates that the analysis would have taken months had he used traditional research methods. But using NLP, he was able to turn the analysis around in only four weeks after receiving the data. He sees this process as having massive untapped potential in the field of educational research.
“There are so many districts that are just sitting on open-ended response data, and NLP can deliver relevant findings in a short amount of time,” says Cox.
Identifying what worked—and what didn’t
The data revealed a trove of information about the unique spring semester. Teachers identified effective practices, such as synchronous learning opportunities to foster positive connections among students, as well as teacher-created instructional videos to deliver content. They also described some barriers to instruction, such as an inability to engage some students over Zoom, disparities in students’ access to technology, and difficulties communicating with parents over email.
The survey also highlighted some unintended consequences of district policies. When students shifted to remote learning in the spring, BPS switched to a no grading policy in an effort to promote equity across the district. But not all teachers saw it that way, says EDC’s Meg Caven, an educational researcher who helped analyze the data.
“The goal of the policy was to guard against the unfair punishment of students by lowering their grades or holding then back a year when structural constraints, rather than effort or merit, influenced students’ ability to learn remotely,” Caven says. “But some teachers’ perception was that the students understood the policy to mean that virtual learning didn’t matter, that their grades wouldn’t change, and that they wouldn’t be held back. Some teachers felt like they had no way to incentivize students to do their classwork.”
Clarkson says that the data on teachers’ perceptions about student engagement was particularly revealing. She notes that many teachers struggled to assess whether or not students were engaged in the lessons—which made sense, since virtual learning environments are so different from face-to-face ones.
“How do you measure student engagement and participation through this technology?” Clarkson asks. “For example, if a student’s camera is off, does that mean that the student is not engaged?”
With the full survey results in hand, Clarkson and the research team are now sharing the findings with district leaders in a series of seminars. One of the topics is expanding the definition of attendance in a virtual environment to better reflect whether students are submitting assignments and logging into class portals during asynchronous learning times. She is also working with the Offices of Instructional Technology and Legal Advisors to understand how to ensure and protect student data privacy while engaging in remote learning.
Within the coming months, Clarkson plans to share the findings with school administrators, teachers, and community members, saying it will help them “understand that we are asking questions, we are listening, and we want to improve practice based on the answers we are getting.”
She also adds that the speed with which Cox completed the analysis shows that educational research can be a vital tool in helping districts adapt to rapidly changing conditions.
“This survey is showing that research isn’t just used for academic purposes,” Clarkson says. “So, how do we leverage that idea, and get research used more frequently—quite literally—in times of crisis?”